Understanding AI Hallucinations: What Are Its Types and How to Prevent It?
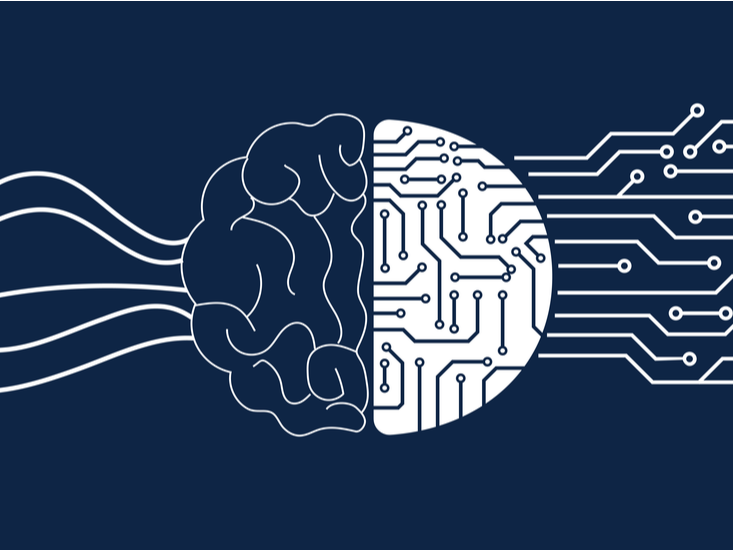
With the increasing prevalence of artificial intelligence (AI) in various industries, people are concerned about AI hallucinations. This happens when AI systems create or understand information that isn’t true, leading to errors and potential dangers.
In this blog, we’ll address AI hallucinations, understand why they occur, and find ways to prevent them. By addressing this issue, we can ensure that AI systems are reliable and trustworthy when they play a bigger role in our world.
Exploring the Types of AI Hallucinations
Let’s explore the different types of AI hallucinations to gain a comprehensive understanding of their nature and implications:
1. Perceptual Hallucinations in AI Systems
AI systems sometimes get things wrong when interpreting or creating false sensory information. This can cause mistakes in computer vision tasks, like recognizing objects in images. These mistakes are called perceptual hallucinations.
They occur because the AI doesn’t have good enough training data or when the algorithms aren’t perfect. Perceptual hallucinations can have different effects, from small errors in recognizing images to large errors in essential systems such as self-driving cars or surveillance systems.
2. Conceptual Hallucinations in AI Systems
AI systems sometimes have conceptual hallucinations, which means they make wrong connections or associations that affect their decision-making. This can lead to giving incorrect or confusing information.
For example, chatbots might provide wrong answers, and recommendation systems could suggest things that don’t make sense. These conceptual hallucinations make AI systems less reliable and helpful.
3. Cognitive Hallucinations in AI Systems
AI can experience cognitive hallucinations that impair its thinking and understanding. This happens when AI systems make incorrect assumptions or predictions based on incomplete or distorted information.
Faulty algorithms, biased training data, or inadequate models can cause these hallucinations. They have already been observed in financial forecasting, risk assessment, and health diagnosis and can lead to incorrect decisions.
Factors Contributing to AI Hallucinations
Several factors contribute to the occurrence of AI hallucinations, leading to distortions and misinterpretations of information generated by AI systems. Some of them are:
1. Insufficient Training Data
AI hallucinations can happen when the AI doesn’t have enough training data. AI learns from the data it’s given to make predictions. If the data is limited or doesn’t have enough variety, the AI might have trouble telling the difference between what’s real and what’s made up.
For example, if the AI is trained with only a few pictures of dogs and sees a picture of a dog it hasn’t seen before, it might make mistakes and not recognize the dog correctly. It might even come up with strange or wrong results.
2. Biased Training Data
Another reason for AI hallucinations is biased training data. AI models can unintentionally learn and repeat biases found in the data they were trained on. If the training dataset is biased, like having more negative portrayals of a certain group compared to others, the AI may produce outputs that reflect these biases.
For instance, if an AI is trained on a biased dataset of news articles, it may generate misleading outputs that strengthen negative stereotypes about a particular group of people.
3. Overconfidence
AI systems can hallucinate if they become too confident. When an AI model thinks it knows everything and is too sure of its abilities, it can produce unreal or pretend results.
This can happen when an AI is trained on a dataset with only correct information but receives incorrect or contradictory inputs to summarize or generate content. The AI’s overreliance on its training data can cause it to produce results that don’t match reality.
How Can AI Hallucinations Be a Problem?
Here’s an elaboration on how AI hallucinations can be problematic:
1. Spreading Misinformation
One big issue with AI hallucinations is that they can spread false information. When an AI creates fake content or unreal outputs, there’s a chance that this false information can be shared with others. This can be harmful because people might make decisions or believe things based on wrong or made-up information.
For example, if an AI is trained on a dataset of fake news articles and generates new articles that seem real, people who read them might unknowingly share and believe the false information. This can lead to the spread of untrue stories and possibly cause harm.
2. Damage to Reputation
AI hallucinations can cause serious harm to the reputation of individuals or organizations by generating embarrassing, offensive, or damaging content.
When an AI like ChatGPT or BardAI produces outputs that include offensive language, harmful stereotypes, or defamatory remarks, it can ruin the reputation of the people or groups mentioned in the content. This can lead to public outrage, loss of trust, and even legal consequences.
For example, suppose an AI trained on social media posts generates offensive or discriminatory content about a particular group. In that case, it can harm the well-being of that group and cause damage to their reputation.
3. Loss of Trust
When AI systems have hallucinations, it can cause people to lose faith in themselves and other AI technologies. If people think AI isn’t unreliable and often provides incorrect or confusing information, they may be hesitant to use AI-supported products or services. This lack of trust can slow the development and diffusion of AI.
For example, suppose many people believe that self-driving cars that use AI are likely to hallucinate and cause accidents. In this case, they’d not trust the technology and would be less willing to use autonomous vehicles. This could hinder the progress and acceptance of self-driving car technology.
How to Prevent AI Hallucinations
Using these strategies and carefully interacting with AI systems can help prevent hallucinations and make AI responses more reliable and accurate.
1. Limit the Possible Outcomes
Limiting what AI can say or do is vital to prevent AI hallucinations. By telling the AI exactly what kind of response you want and limiting the choices, you reduce the likelihood that the AI will give incorrect or silly information.

For example, when talking to the AI, you can ask it for a simple “yes” or “no” or choose an answer from a list you’ve already provided. By giving clear instructions and setting boundaries, you leverage the AI’s knowledge and get it to give accurate and specific answers.
2. Pack in Relevant Data and Sources Unique to You
You must give the AI system specific and relevant information about your situation so that the AI responds more accurately and helpfully. Instead of giving general prompts, you should provide details, facts, and data points relevant to your topic.
Giving the AI relevant information gives it more context and helps it respond better. This reduces the risk of hallucinations and ensures that the AI better understands and answers your problem or question.
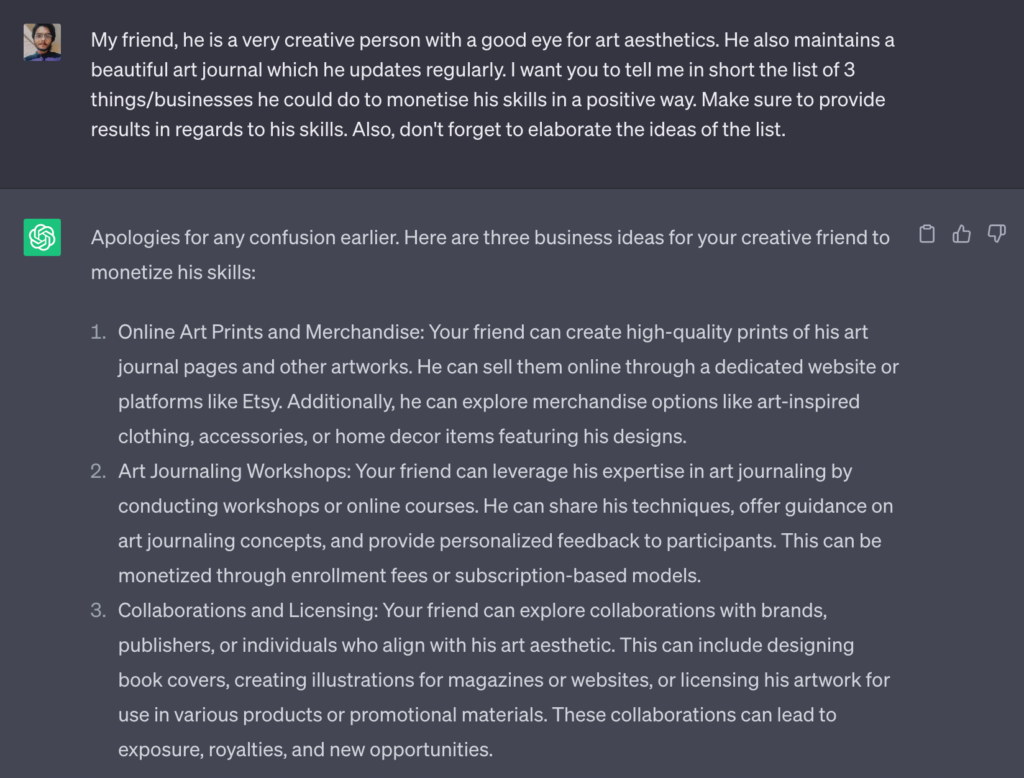
3. Create a Data Template for the Model to Follow
If you have calculations or tasks that rely on data, you can give the AI a data template to avoid errors and hallucinations. Instead of just using text, you should create a table or structure that shows the AI how to handle the data.
A format that the AI can easily understand clarifies things and helps it perform accurate calculations or analyses. This way, you reduce the likelihood of wrong answers and guide the AI to a more reliable result.
4. Give the AI a Specific Role—and Tell It Not to Lie
Giving specific roles to the AI helps give the AI a clear mission and tell it not to lie to avoid hallucinations. You tell the AI how to behave by giving it a specific role, such as a math expert or a history guide. This helps the AI focus on providing correct information for its assigned task and reduces the likelihood of making up incorrect responses.
It’s also important to explicitly tell the AI to admit when it doesn’t know something rather than make something up. This makes the AI more honest and keeps it from spreading false information.
5. Tell It What You Want—and What You Don’t Want
To prevent receiving unwanted information from the AI, you can control its behavior in advance by anticipating its response. Make it clear in your prompt what you want and don’t want by giving clear instructions to the AI.
For example, you can ask only for factual information or the AI to avoid specific results. Setting these boundaries helps the AI provide more accurate and relevant answers and reduce the likelihood of hallucinations.
In Summary
It’s essential to address AI hallucinations to build trustworthy and reliable AI systems in our world that increasingly rely on AI. We can do this by knowing why they occur and taking steps to prevent them.
Ways to prevent AI hallucinations include limiting what the AI can do, giving it the correct information, using data templates, assigning specific tasks, setting limits, and trying different settings. In this way, we improve the reliability and accuracy of AI systems. Preventing AI hallucinations ensures that AI technologies work safely and effectively in various domains.
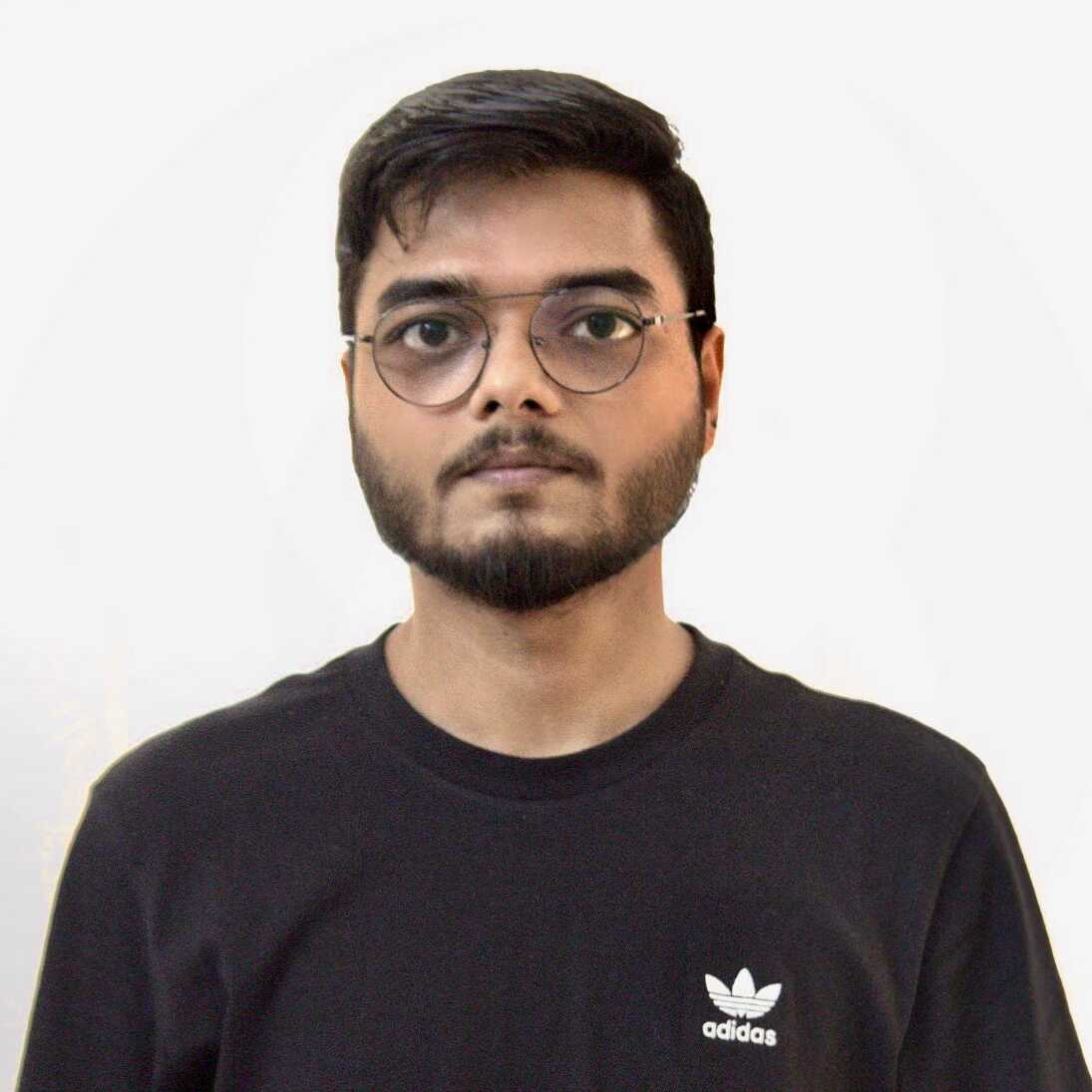
I’m an AI enthusiast who loves to scour the world of Artificial Intelligence and share the latest updates with you. I’m all about exploring the mind-boggling potential of tech, especially the mystical realm of Web 3.0. When I’m not geeking out, you’ll find me capturing fleeting moments or experimenting with new recipes that may or may not be edible.